Machine Learning in Trading: A Cutting-Edge Approach to Profitability
- November 3, 2024
- Posted by: Drglenbrown1
- Category: Machine Learning in Trading
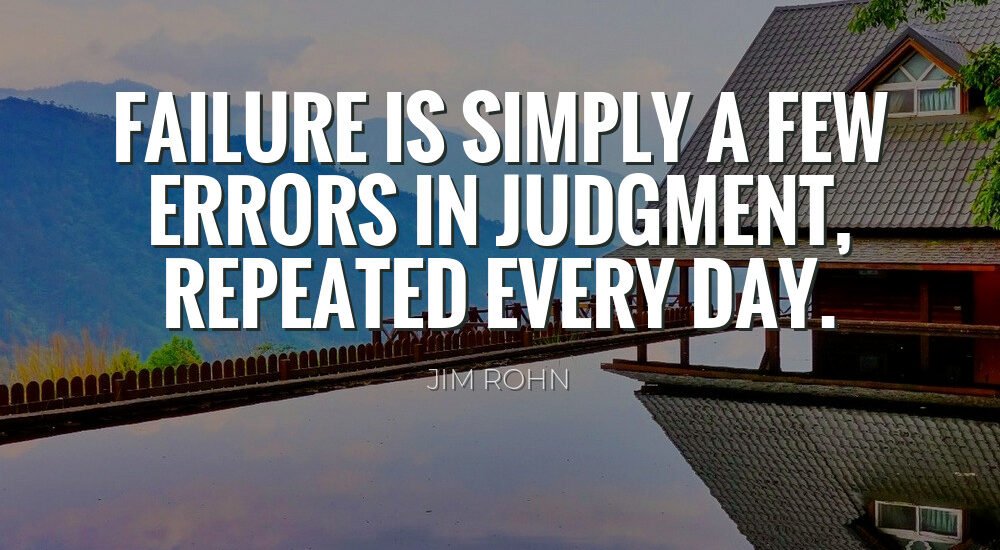
In today’s financial landscape, machine learning (ML) has become an essential tool for traders seeking a competitive edge. This article examines the power of machine learning in trading, exploring its applications, benefits, and how it shapes the future of proprietary trading.
The Role of Machine Learning in Financial Markets
Machine learning in trading utilizes algorithms to analyze vast amounts of data, detect patterns, and make predictions with minimal human intervention. For proprietary traders, ML offers critical advantages, including:
- Predictive Analytics: Machine learning algorithms analyze historical and real-time data to identify patterns and forecast future price movements.
- Risk Management: By analyzing factors like volatility and market sentiment, ML models enhance risk assessment, allowing traders to set adaptive risk parameters.
- Automated Trading Systems: ML algorithms power autonomous trading systems that execute trades at speeds and accuracy levels beyond human capacity, crucial for high-frequency and algorithmic trading.
Key Applications of Machine Learning in Trading
- Pattern Recognition: Identifying trends, reversals, and market sentiment through pattern recognition enables traders to anticipate changes in price direction.
- Sentiment Analysis: Algorithms can analyze news articles, social media, and financial reports, providing insights into market sentiment that might affect asset prices.
- Portfolio Optimization: ML models optimize portfolio allocation by balancing risk and return based on real-time and historical data.
- Anomaly Detection: Machine learning detects unusual trading patterns, which can signal fraudulent activities or unforeseen market events.
Types of Machine Learning Models Used in Trading
- Supervised Learning: Algorithms are trained on historical data with labeled outcomes, helping traders forecast asset prices based on past trends.
- Unsupervised Learning: These models identify patterns in data without labeled outcomes, useful in clustering assets or detecting unusual price behaviors.
- Reinforcement Learning: Algorithms learn optimal trading actions by interacting with market environments, adjusting strategies to maximize rewards over time.
Challenges and Considerations in Machine Learning for Trading
- Data Quality and Availability: Machine learning is only as good as the data it’s trained on. Ensuring high-quality, diverse datasets is crucial.
- Overfitting: Overly complex models may fit historical data perfectly but fail in live markets. Regular validation helps maintain model accuracy.
- Regulatory Compliance: Proprietary traders must ensure their ML-driven strategies comply with trading regulations, particularly in high-frequency and algorithmic trading.
- Interpretability: Black-box models may lack transparency, so traders need methods to interpret model predictions, ensuring reliable and accountable trading decisions.
Future of Machine Learning in Proprietary Trading
As technology advances, machine learning is poised to play an even more significant role in trading. Emerging trends include:
- Integration with Quantum Computing: Combining ML with quantum computing could exponentially increase processing power, enabling models to analyze even larger datasets in real time.
- AI-Driven Strategy Development: As ML models evolve, they could autonomously develop, test, and implement new trading strategies based on continuous learning from market dynamics.
- Improved Risk Management Tools: ML-driven tools are expected to provide traders with more precise and adaptive risk management strategies, adjusting automatically to market conditions.
About the Author
Dr. Glen Brown, Ph.D. in Investments and Finance, is a leader in integrating machine learning and algorithmic trading strategies. As President & CEO of Global Financial Engineering, Inc., he brings over 25 years of experience in finance and innovation, empowering traders through data-driven insights and cutting-edge financial technology.
General Disclaimer
The educational content provided in this article is for informational purposes only and does not constitute financial advice or recommendations. Trading and investing in financial markets involve significant risk. Always conduct your research or consult a professional before making trading decisions.