The Evolution of Financial Engineering: From Theory to Systematic Trading
- April 2, 2025
- Posted by: Drglenbrown1
- Category: Financial Engineering
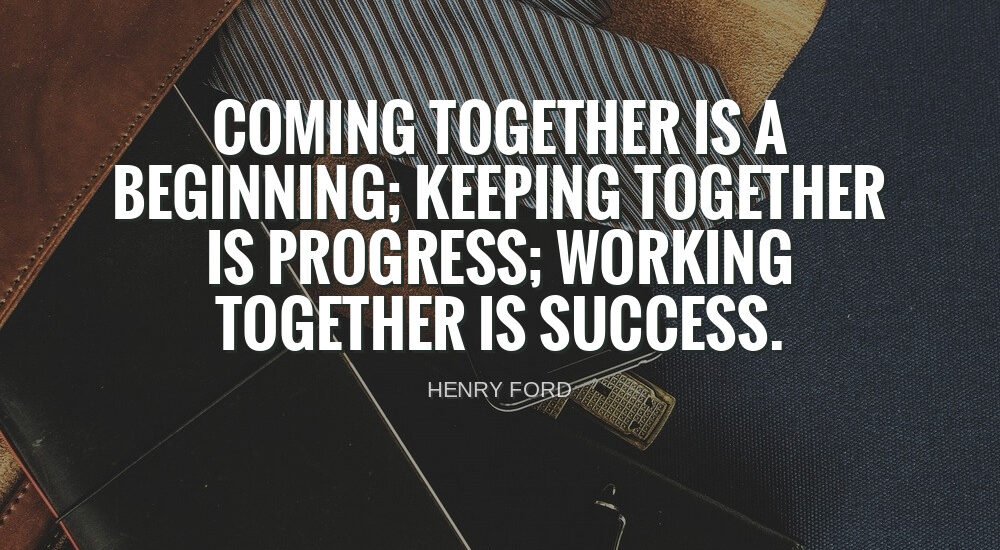
Financial engineering has undergone a remarkable transformation over the past few decades. What began as a largely academic field—dominated by theoretical models and mathematical constructs—has evolved into a dynamic discipline that underpins some of today’s most advanced systematic trading strategies. This evolution reflects a journey from pure theory to practical application, driven by technological advancements, data availability, and the relentless pursuit of improved risk management.
1. The Origins of Financial Engineering
Academic Foundations
Financial engineering originally emerged from academic research in the 1960s and 1970s, drawing on principles from mathematics, statistics, economics, and computer science. Early pioneers developed models for pricing options and other derivatives, with seminal work such as the Black-Scholes-Merton model laying the groundwork for modern derivatives pricing.
- Theoretical Models:
Early models were designed to solve complex problems—like determining the fair value of options—using stochastic calculus and other mathematical techniques. - Academic Rigor:
These models were often confined to research papers and academic circles, where the focus was on theoretical elegance and mathematical precision.
Bridging the Gap
Despite their brilliance, these early models had limitations in practical application. Financial markets are complex, dynamic, and influenced by myriad factors that static models struggle to capture. Over time, practitioners began to recognize that to truly harness the power of these models, they needed to be adapted into systems that could operate in real time, account for market noise, and adjust to evolving risk conditions.
2. Technological Advancements and the Rise of Systematic Trading
The Digital Revolution
The advent of high-speed computing and sophisticated data analytics revolutionized the financial markets. With the ability to process vast amounts of data in real time, financial engineers began developing automated trading systems that could implement and adjust complex models on the fly.
- Algorithmic Trading:
Systems emerged that could execute trades based on pre-determined criteria, continuously monitoring market conditions and making split-second decisions. - Data-Driven Insights:
The integration of big data analytics allowed these systems to refine trading signals, filter out noise, and optimize risk management.
From Theory to Practice
The transformation from academic theory to practical application is epitomized by frameworks such as our Global Algorithmic Trading Software (GATS). This framework takes established theoretical constructs—like the Average True Range (ATR), dynamic risk management principles, and statistical measures—and integrates them into a cohesive, automated trading system.
- Adaptive Risk Management:
GATS employs the Dynamic Adaptive ATR Trailing Stop (DAATS) mechanism, which dynamically adjusts stop-loss levels based on current market volatility. This approach exemplifies how theory (in this case, volatility measurement and adaptive scaling) is turned into a practical tool. - Multi-Timeframe Analysis:
By leveraging multiple timeframes, the system validates trading signals with higher-level trend governors (like the Daily MACD), ensuring that trades are executed only when aligned with the broader market trend. - Statistical Foundations:
The integration of statistical measures—such as the standard deviation of volatility and variance analysis—ensures that the system’s risk controls are both adaptive and robust, thereby enhancing overall performance.
3. Systematic Trading in Practice
Building an “ATM-like” Trading System
The evolution of financial engineering is not only a matter of academic interest; it has practical implications for building high-performance trading systems. Our goal is to develop an “ATM-like” trading system—one that operates with near-perfect efficiency and consistently delivers superior risk-adjusted returns.
- Proprietary Strategies:
Our system deploys multiple proprietary strategies across different asset classes and timeframes, ranging from ultra-short-term scalping in forex to long-term trend following in digital currencies. - Dynamic Exits and Position Additions:
A key element of our methodology is the ability to adapt exit strategies dynamically. For instance, our DAATS-based trailing stops adjust in real time, securing unrealized profits and allowing additional positions to be layered on as market conditions evolve. - Risk and Capital Management:
We convert capital into discrete units—each valued at US$1.00—to standardize risk management and performance tracking. This unit-based system ensures transparency, consistent position sizing, and scalable risk control across the entire portfolio.
Real-World Impact
Systematic trading systems, grounded in robust financial engineering, have become a cornerstone of modern financial markets. By continuously refining our models and integrating adaptive technologies, we can:
- Capture Market Inefficiencies:
Exploit opportunities that arise from market noise and transient inefficiencies. - Enhance Risk-Adjusted Returns:
Improve performance metrics such as the Sharpe ratio through dynamic risk management. - Ensure Consistency:
Maintain discipline and consistency in trade execution, regardless of market volatility.
4. Conclusion
The evolution of financial engineering—from its academic origins to its current role as the backbone of systematic trading—illustrates the power of bridging theory with practice. Our Global Algorithmic Trading Software (GATS) exemplifies this journey by integrating adaptive risk management, multi-timeframe analysis, and dynamic position management into a single, robust framework.
This transformation is not merely about implementing models; it’s about continuously refining them to create a trading system that is adaptive, efficient, and capable of delivering superior risk-adjusted returns. As we move forward, our commitment to innovation in financial engineering will remain the driving force behind our success, ensuring that our approach evolves with the markets and continues to set new industry standards.
About the Author
Dr. Glen Brown is a pioneer in financial engineering and algorithmic trading. With decades of experience bridging academic theory with practical applications, Dr. Brown is the visionary founder of Global Accountancy Institute, Inc. and Global Financial Engineering, Inc. His innovative GATS Framework has set new industry standards in adaptive risk management and multi-timeframe analysis, driving consistent, superior trading performance.
General Disclaimer
The information presented in this paper is for educational and informational purposes only and should not be construed as investment advice. Trading in financial markets involves risk, and past performance is not indicative of future results. Readers are encouraged to conduct their own research and consult with a qualified financial advisor before making any investment decisions.
Global Accountancy Institute, Inc. and Global Financial Engineering, Inc. operate as closed proprietary firms. We do not offer any products or services to the general public, nor do we accept clients or external funds. All methodologies, including the GATS Framework, are exclusively developed and utilized internally as part of our proprietary trading systems.
Neither Dr. Glen Brown nor his affiliated institutions accept any responsibility for any loss or damage incurred as a result of the use or application of the information provided.